Comprehensible AI decisions
Relevance
Most people use or interact with AI on a daily basis without actively realizing it. Be it the chatbot for customer service or automatic facial recognition on smartphones. Incorrect or faulty AI decisions are annoying in these cases, but without serious consequences for the user. However, such uncertainties are not acceptable in many areas of application, such as production or autonomous driving. This is because an incorrect decision by the AI system could potentially pose a direct risk to humans in these areas. Therefore, the highest demands must be placed on the AI in terms of accuracy and stability. As a result, explainable artificial intelligence (XAI) methods must be used during the development of such systems so that an AI is not only evaluated on the basis of metrics such as accuracy, but developers can also directly understand how decisions are made. For example, it is possible to identify whether the AI is exploiting an error or unwanted structures in the training data. However, it is not only interesting for experts to understand the behavior of an AI, but also for end users - in some situations even necessary. Under the General Data Protection Regulation (GDPR), data subjects already have a right to transparency and explanation. In the case of an AI system, this can only be guaranteed through explainable and comprehensible AI decisions due to the black box problem. Further regulations, such as the draft AI Regulation (KI-VO-E), could impose additional legal obligations regarding explainable AI in the future.
In addition to legal reasons, ethical reasons are also relevant for the use of XAI. In this context, the EU Commission presented "Ethics guidelines for trustworthy AI", which provide developers with seven key requirements for AI systems that must be observed. In general, according to these guidelines, an AI should support users in making decisions, but never make a decision about a human being itself. This is intended to ensure that people always have decision-making authority over technology. Taking the financial sector as an example, the aim is to prevent discrimination against individual groups of people when granting loans. XAI can help build trust in AI systems and empower people to make informed decisions.
Objective
KARL aims to compile the current state of the art on XAI methods and identify the relevant information that makes an AI-based decision comprehensible to humans. To this end, human-machine interaction in explainable AI systems will be investigated using experimental studies. A series of expert interviews will also be used to identify potential for the use of XAI in the manufacturing industry. Various use cases will be used to investigate when XAI can help to increase the benefits of AI and minimize the risks associated with its use.
The results of the research on the state of the art are prepared in the form of an XAI training course and supplemented by the findings from the experimental studies and the potential analysis. The training content will not only be available on the KARL website, but will also be offered to interested parties in the form of an interactive workshop.
Specifically, KARL would like to answer the following questions:
- What are the reasons for using XAI? Are there legal and ethical necessities?
- Which XAI methods exist and when can they be used?
- What are the special features, advantages and disadvantages of individual XAI methods?
- How can AI decisions be made comprehensible for humans?
The focus will be on the application of XAI for end users.
Handouts for companies
- XAI online course (course to introduce the motivation behind XAI, the reasons for using XAI, the different target groups of an explanation, important concepts and concrete XAI methods)
- XAI workshop offer (face-to-face event to impart basic knowledge on explainable AI using illustrative use cases, interactive demonstrators and exercises)
- XAI selection system (tool for identifying suitable XAI methods for your own AI use case)
Comprehensible AI decisions
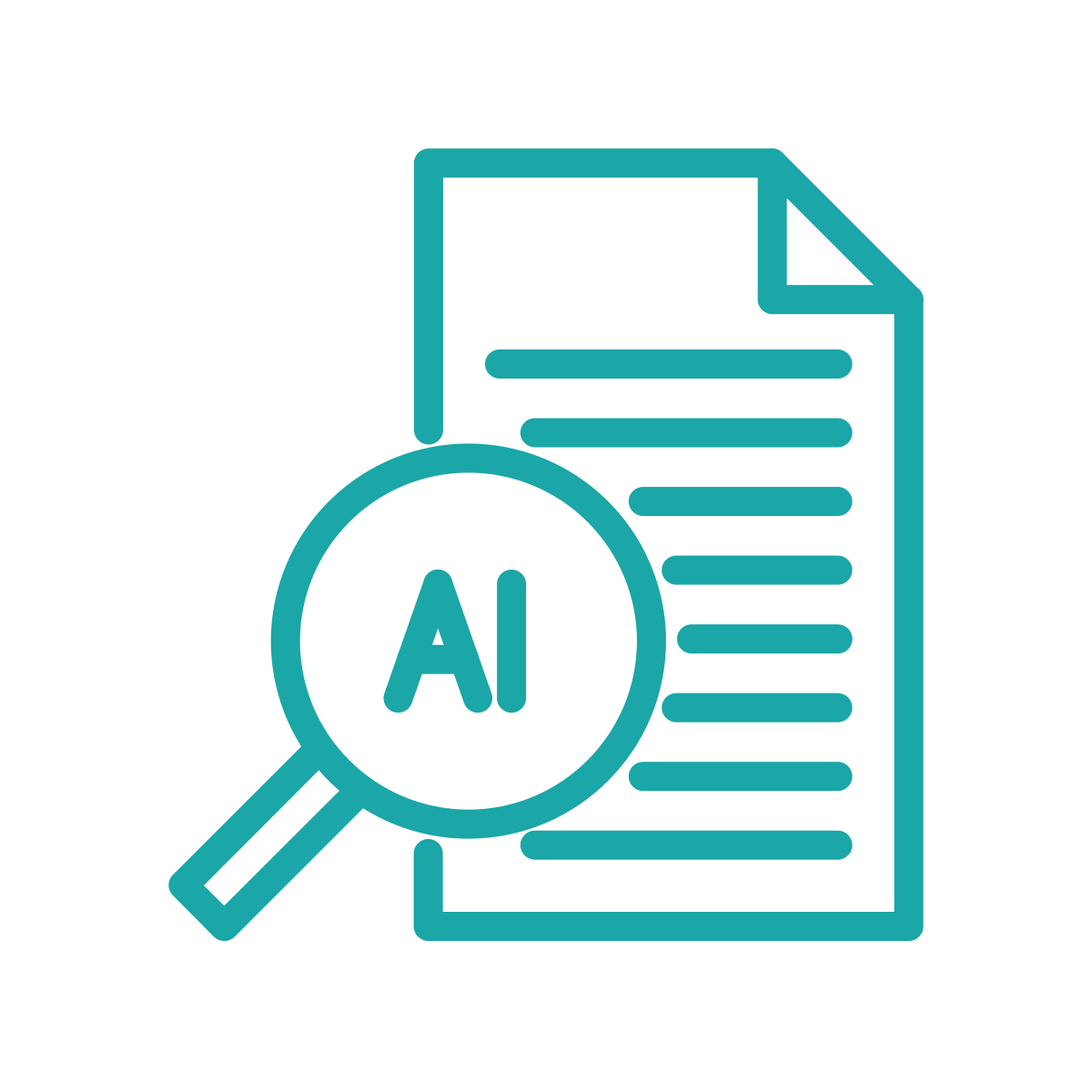
Relevance
Most people use or interact with AI on a daily basis without actively realizing it. Be it the chatbot for customer service or automatic facial recognition on smartphones. Incorrect or faulty AI decisions are annoying in these cases, but without serious consequences for the user. However, such uncertainties are not acceptable in many areas of application, such as production or autonomous driving. This is because an incorrect decision by the AI system could potentially pose a direct risk to humans in these areas. Therefore, the highest demands must be placed on the AI in terms of accuracy and stability. As a result, explainable artificial intelligence (XAI) methods must be used during the development of such systems so that an AI is not only evaluated on the basis of metrics such as accuracy, but developers can also directly understand how decisions are made. For example, it is possible to identify whether the AI is exploiting an error or unwanted structures in the training data. However, it is not only interesting for experts to understand the behavior of an AI, but also for end users - in some situations even necessary. Under the General Data Protection Regulation (GDPR), data subjects already have a right to transparency and explanation. In the case of an AI system, this can only be guaranteed through explainable and comprehensible AI decisions due to the black box problem. Further regulations, such as the draft AI Regulation (KI-VO-E), could impose additional legal obligations regarding explainable AI in the future.
In addition to legal reasons, ethical reasons are also relevant for the use of XAI. In this context, the EU Commission presented "Ethics guidelines for trustworthy AI", which provide developers with seven key requirements for AI systems that must be observed. In general, according to these guidelines, an AI should support users in making decisions, but never make a decision about a human being itself. This is intended to ensure that people always have decision-making authority over technology. Taking the financial sector as an example, the aim is to prevent discrimination against individual groups of people when granting loans. XAI can help build trust in AI systems and empower people to make informed decisions.
Objective
KARL aims to compile the current state of the art on XAI methods and identify the relevant information that makes an AI-based decision comprehensible to humans. To this end, human-machine interaction in explainable AI systems will be investigated using experimental studies. A series of expert interviews will also be used to identify potential for the use of XAI in the manufacturing industry. Various use cases will be used to investigate when XAI can help to increase the benefits of AI and minimize the risks associated with its use.
The results of the research on the state of the art are prepared in the form of an XAI training course and supplemented by the findings from the experimental studies and the potential analysis. The training content will not only be available on the KARL website, but will also be offered to interested parties in the form of an interactive workshop.
Specifically, KARL would like to answer the following questions:
- What are the reasons for using XAI? Are there legal and ethical necessities?
- Which XAI methods exist and when can they be used?
- What are the special features, advantages and disadvantages of individual XAI methods?
- How can AI decisions be made comprehensible for humans?
The focus will be on the application of XAI for end users.
Handouts for companies
- XAI online course (course to introduce the motivation behind XAI, the reasons for using XAI, the different target groups of an explanation, important concepts and concrete XAI methods)
- XAI workshop offer (face-to-face event to impart basic knowledge on explainable AI using illustrative use cases, interactive demonstrators and exercises)
- XAI selection system (tool for identifying suitable XAI methods for your own AI use case)
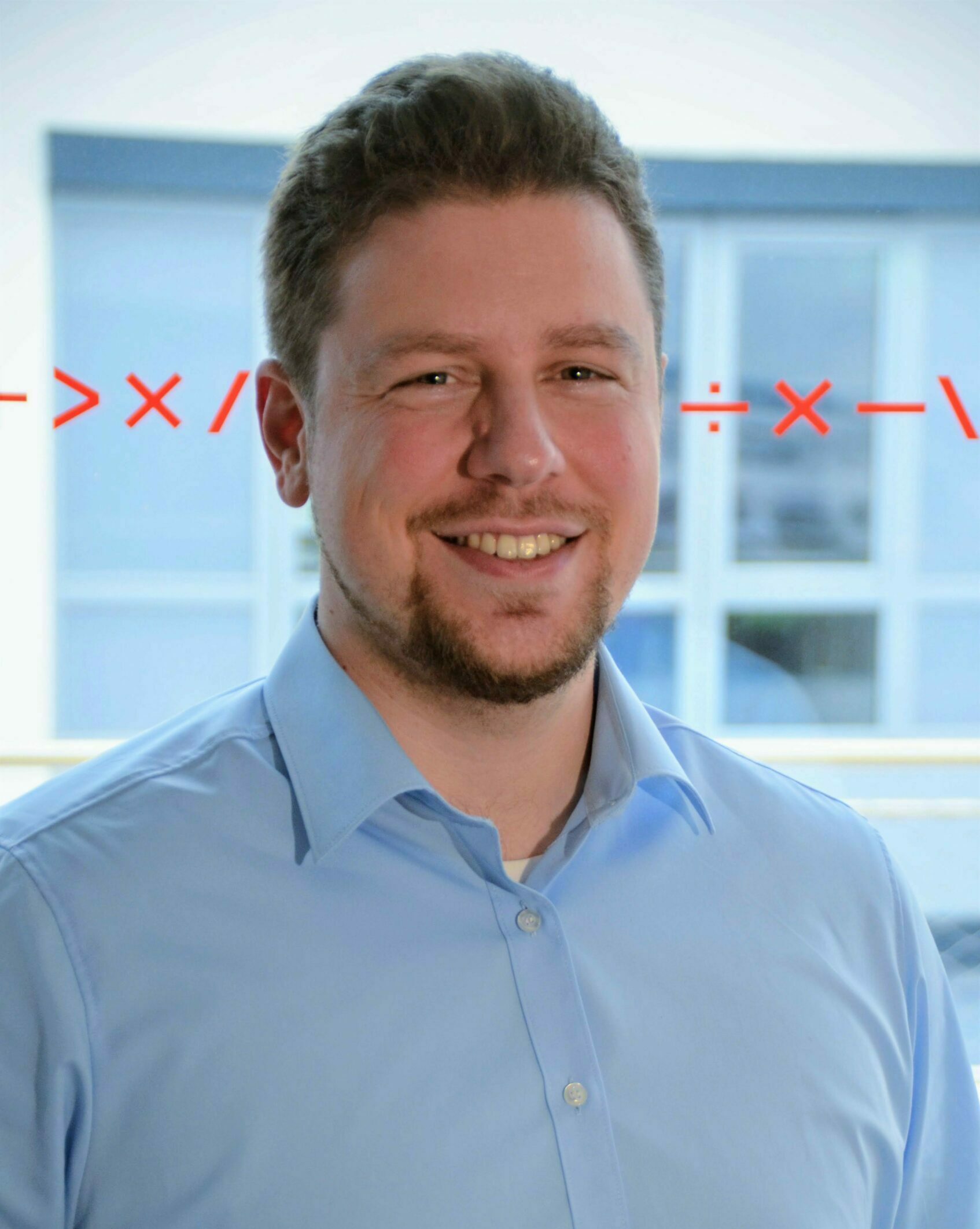
Robin Weitemeyer
Karlsruhe University of Applied Sciences
robin.weitemeyer@h-ka.de
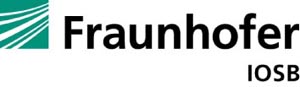
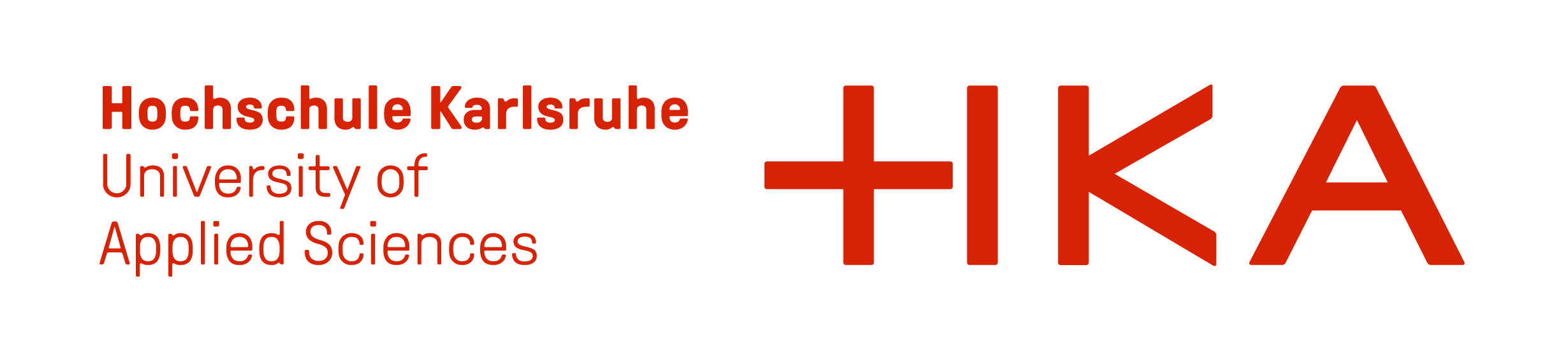
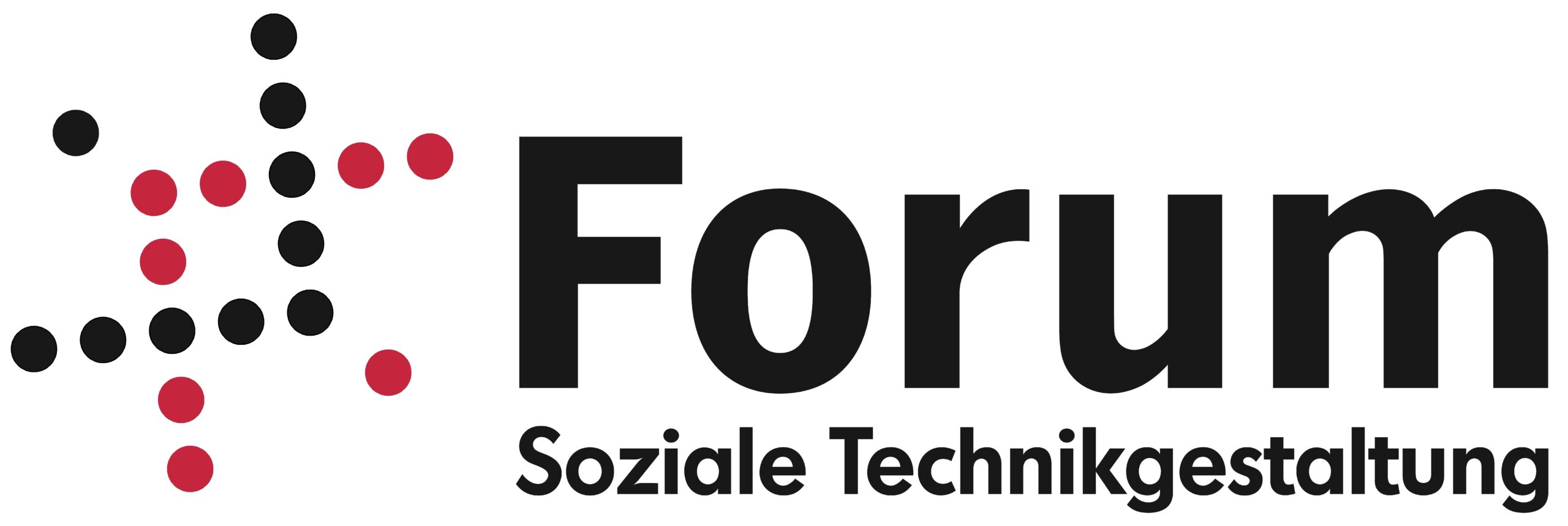
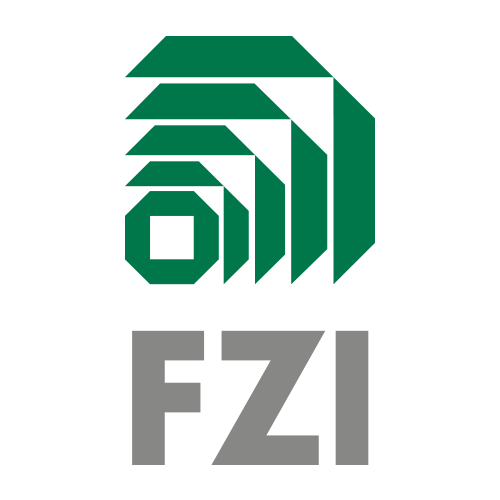
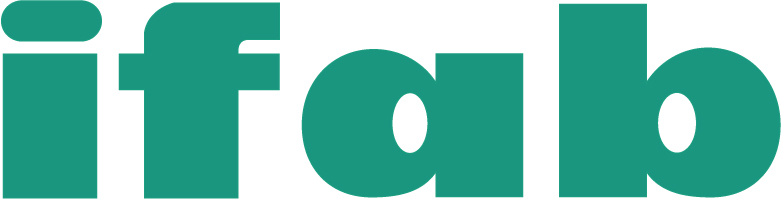
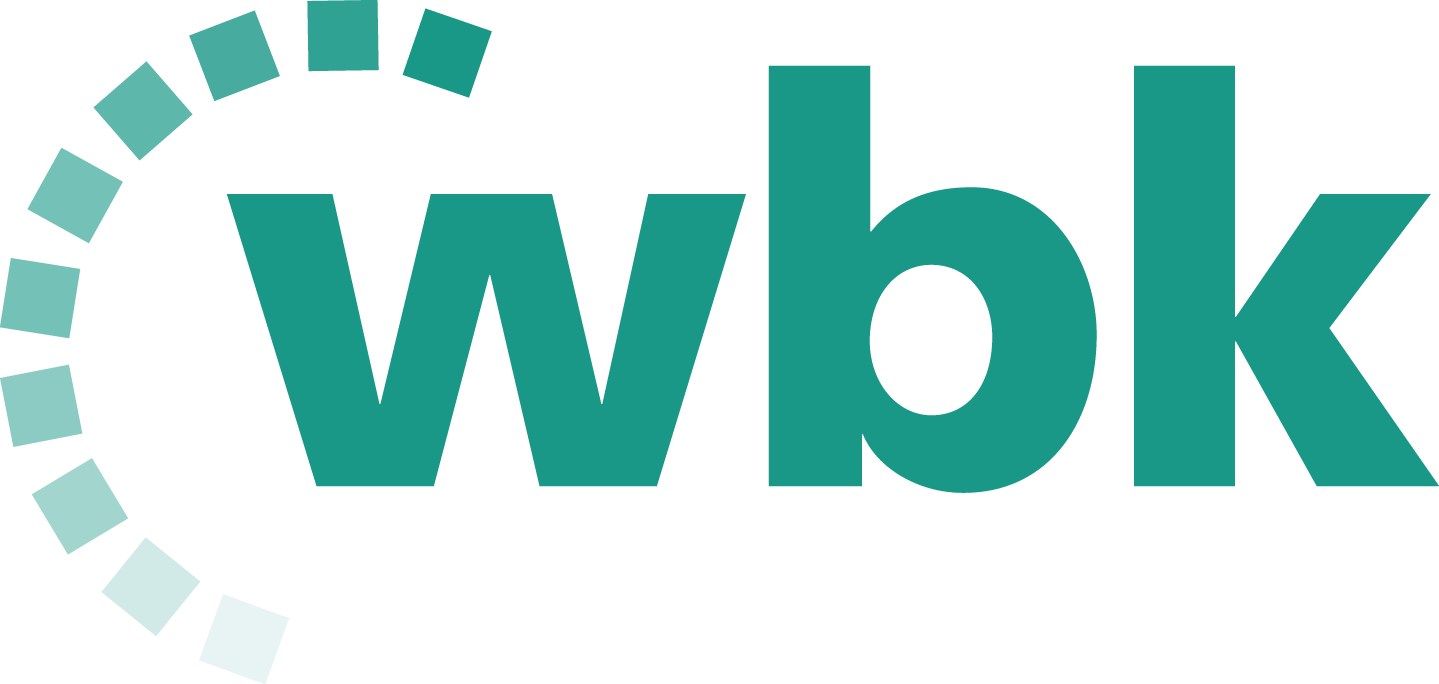
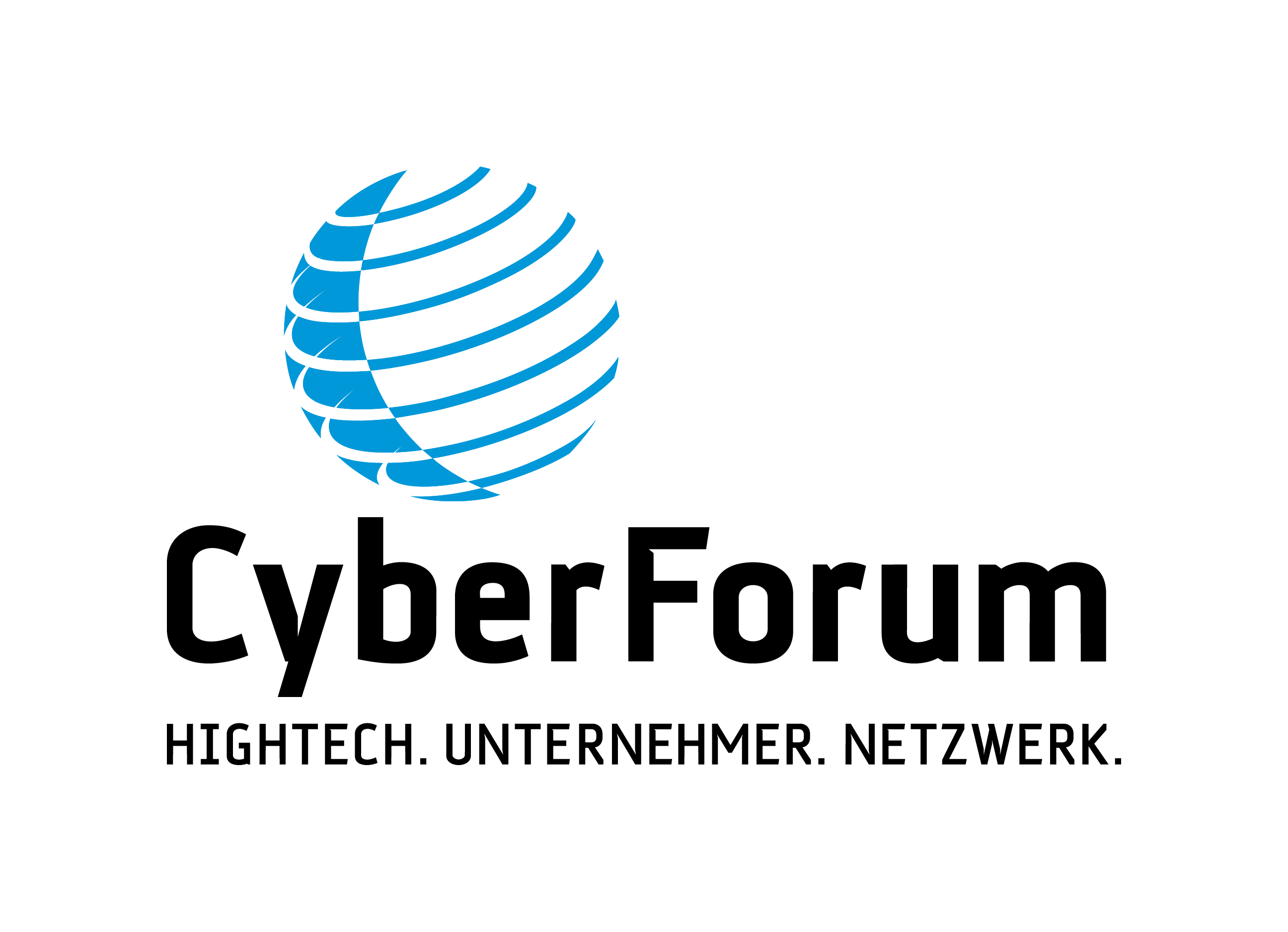