Machine learning based on secret and/or personal data
Get to know background information and approaches for technically securing the training and operation of artificial intelligence (AI) models: What measures can be taken at the level of data (privacy enhancing technologies, anonymization) and architecture (distributed learning, trusted computing)? What are the advantages and limitations of the different options?
Target group
The overview document is aimed at project managers, data scientists and developers who want to deal with information security issues.
Expected result
The document provides a basic understanding of IT security and privacy issues relating to the use of machine learning methods as well as corresponding solution approaches. It provides an overview of the assets to be protected when using ML on the basis of sensitive data, which architectures and technical approaches can be considered to protect sensitive data in training and/or in the operation of AI systems and how relevant the individual approaches are in practice. Privacy attacks on AI models, which are currently still the subject of research, are also presented. These show the relevance of information security in the use of AI in the future.
General conditions
No special requirements.
Operating instructions
Not necessary.
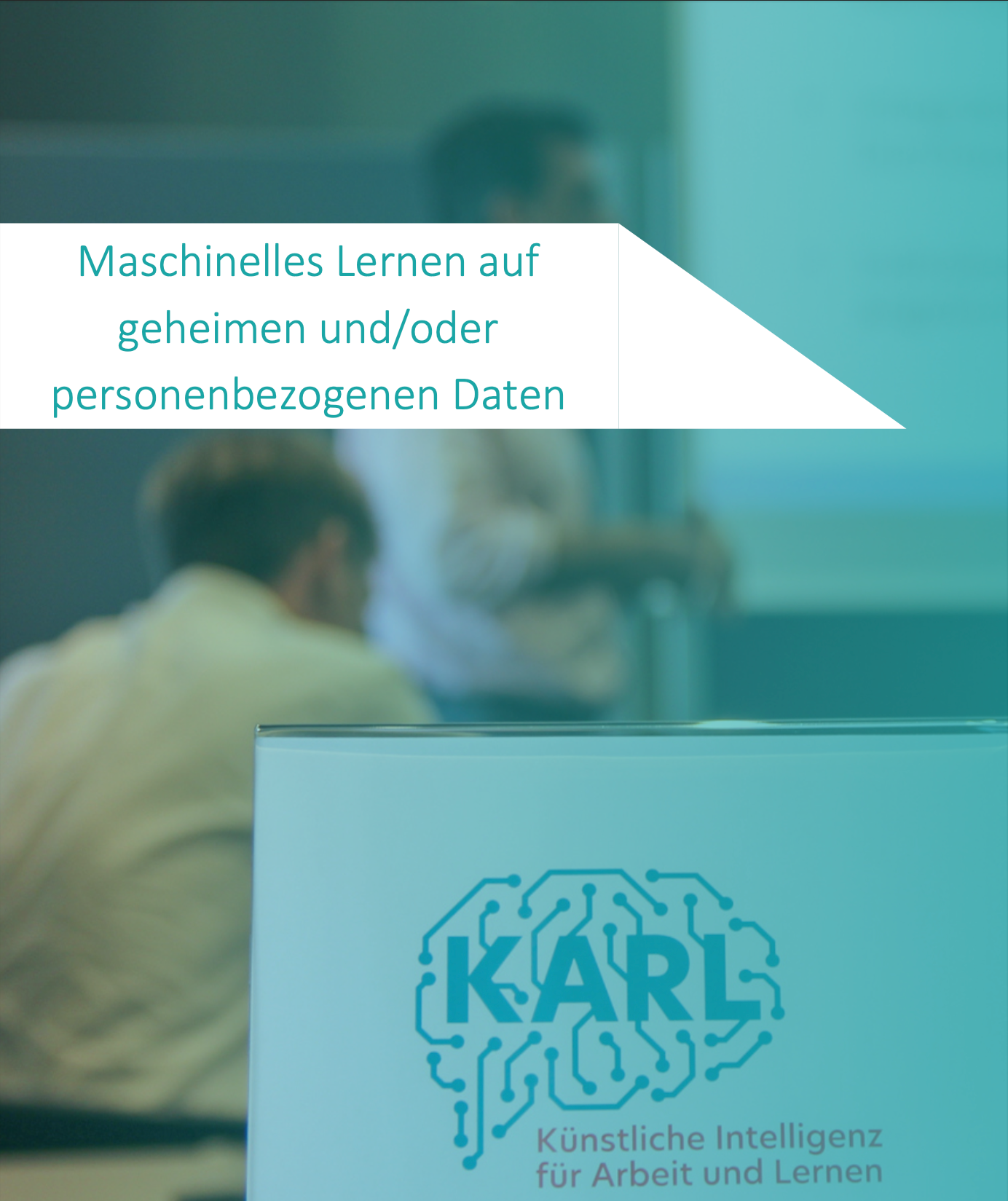
Contact person
Dr. Pascal Birnstill | Fraunhofer IOSB
pascal.birnstill@iosb.fraunhofer.de
pascal.birnstill@iosb.fraunhofer.de
Format
PDF, service
Machine learning based on secret and/or personal data
Get to know background information and approaches for technically securing the training and operation of artificial intelligence (AI) models: What measures can be taken at the level of data (privacy enhancing technologies, anonymization) and architecture (distributed learning, trusted computing)? What are the advantages and limitations of the different options?
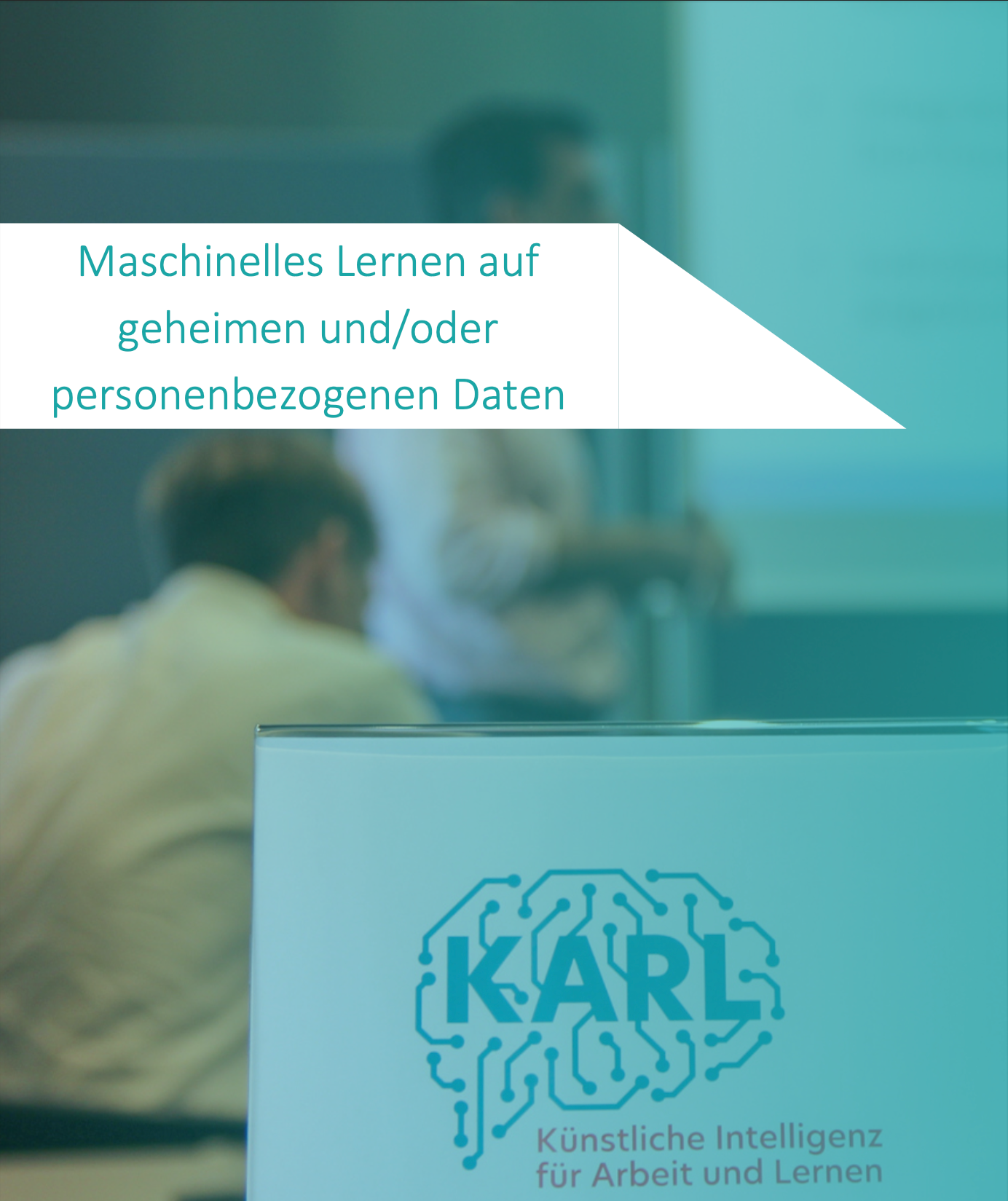
Target group
The overview document is aimed at project managers, data scientists and developers who want to deal with information security issues.
Expected result
The document provides a basic understanding of IT security and privacy issues relating to the use of machine learning methods as well as corresponding solution approaches. It provides an overview of the assets to be protected when using ML on the basis of sensitive data, which architectures and technical approaches can be considered to protect sensitive data in training and/or in the operation of AI systems and how relevant the individual approaches are in practice. Privacy attacks on AI models, which are currently still the subject of research, are also presented. These show the relevance of information security in the use of AI in the future.
General conditions
No special requirements.
Operating instructions
Not necessary.
Contact person
Dr. Pascal Birnstill | Fraunhofer IOSB
pascal.birnstill@iosb.fraunhofer.de
pascal.birnstill@iosb.fraunhofer.de
Format
PDF, service